UCLA GailBot Hackathon Weekend
GailBot: The Challenges of Internationalizing, Scaling Up, and Working With Other People’s Data
GailBot is a new transcription software system that combines automatic speech recognition (ASR), machine learning (ML) models, and research-informed heuristics to capture often-overlooked paralinguistic features such as overlaps, sound stretches, speed shifts, and laughter. Over the last six months, the GailBot development team, based at Tufts University in Boston and Loughborough University in the UK—with the support of the SAGE concept grant—has been working on rebuilding GailBot into a more widely accessible tool for researchers. This new version of GailBot features a new graphical user interface (GUI), additional features based on beta user feedback, and a new plugin architecture to enable sustainable scaling and growth as ML models, annotated training data, and ML tools become more accessible.
In this blog post, we will report on the team’s recent week-long hack session at UCLA, outlining how we are adapting GailBot in response to user feedback and our longer-term goals for scaling up the tool.
A Hack-session at the Birthplace of Conversation Analysis
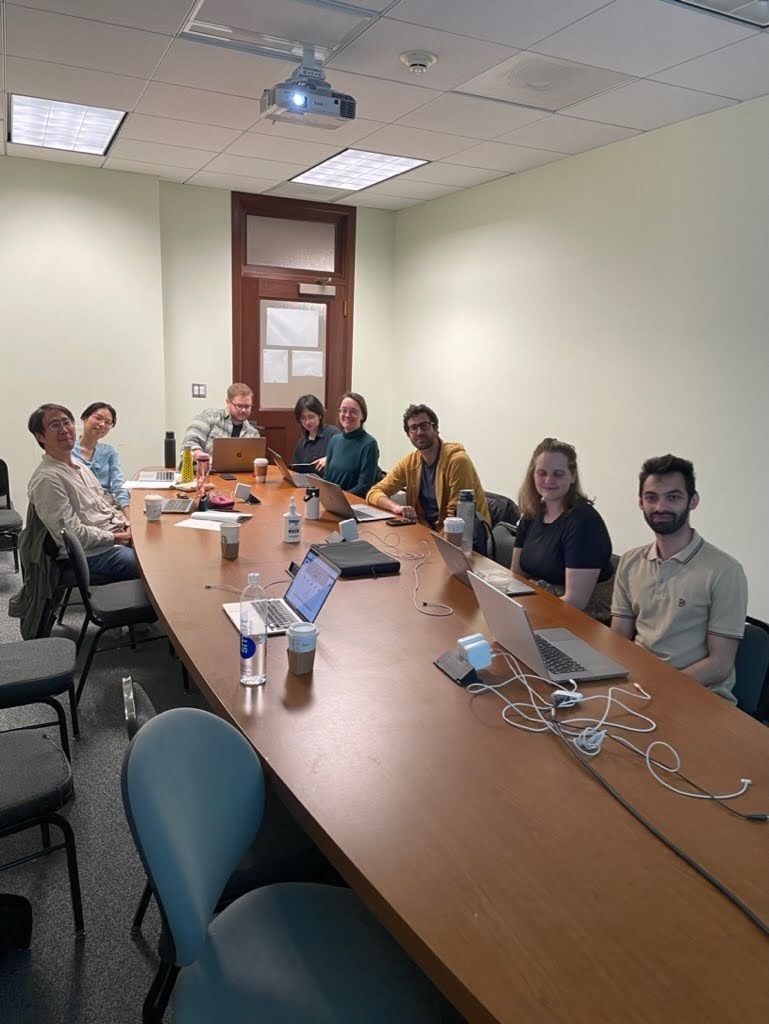
In April 2024, four members of the GailBot team (Muhammad Umair, Vivian Li, Saul Albert, Julia Mertens, and J.P. De Ruiter) gathered in the basement of Haines Hall at UCLA for an intense week of work on GailBot, collaborating with researchers from UCLA’s Conversation Analysis Working Group. GailBot is named after Gail Jefferson, who invented the detailed system of transcription used in the field of Conversation Analysis (CA) while she was an undergraduate at UCLA, making this venue particularly fitting. The hack-session ran through the weekend and involved a highly committed group of staff, graduate students, and visiting scholars from around the world.
Working With Other People’s Data
One of the significant challenges during the session was working with diverse data sets. GailBot was initially developed to address a specific research problem at the Human Interaction Lab at Tufts: how to transcribe hundreds of hours of high-quality, neatly diarized video recordings of dialogue in US English without incurring prohibitive costs or logistical difficulties. However, many participants had data sets that were far more challenging to work with. For instance, not all recordings had neatly separated audio channels for each speaker, requiring us to develop basic functional workflows for different types of recordings.
Internationalization
We also worked with experts on Japanese, Cantonese, and Mandarin conversational data. ASR for these languages often does not work as well as it does for English, and in the case of minority languages such as Cantonese, ASR models are not available in the four ASR engines GailBot currently uses (IBM Watson, OpenAI Whisper/WhisperX, Google). We had to experiment with many combinations and perform considerable hacking to achieve usable results.
GailBot’s Next Steps
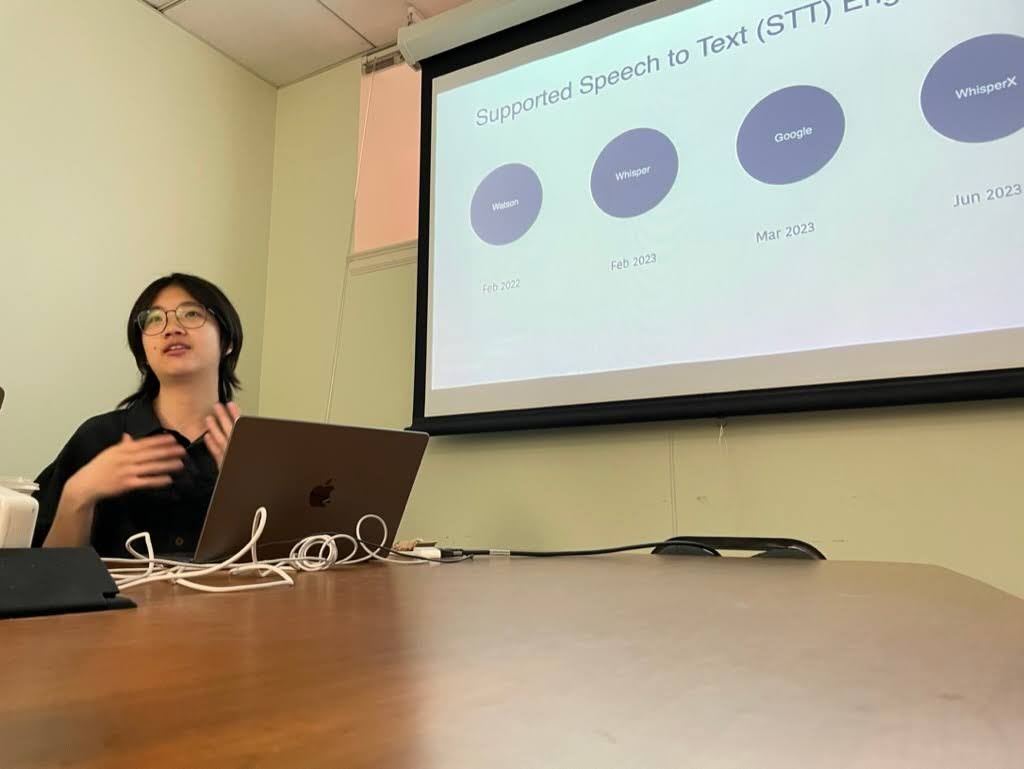
We concluded our workshop by prioritizing features that the participants identified as critical for promoting GailBot to their colleagues:
- Create multi-format outputs for digital transcription tools like CLAN, ELAN, and EXMARaLDA.
- Prevent the ASR systems in GailBot from ‘helpfully’ cleaning up transcripts.
- Enable users to dynamically configure all features of the ASR engines through GailBot.
Since returning from UCLA, we have set up a Slack Community to stay in touch with our growing community of beta testers. You can join this community on the GailBot site, where you can also download beta versions of GailBot for Mac OS, read documentation, and watch how-to videos.
Learn more about GailBot and join our community to stay updated on our progress and contribute to the development of this powerful tool for conversation analytic research.